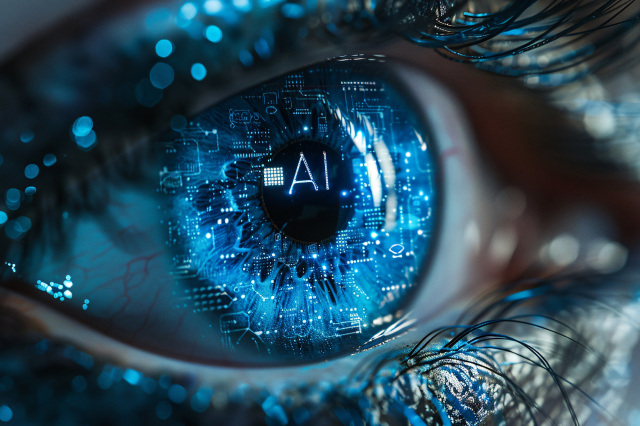
Causal Inference and Discovery:
Elevating Recommendation Systems for Smarter AI
Our ongoing mission is to push the boundaries of artificial intelligence to build more innovative, more efficient systems across a wide range of sectors, from connected vehicle systems and V2X architecture to telecommunications and smart cities. As AI continues to evolve, one of the most promising innovations is the integration of causal inference and causal discovery methods to create more accurate and adaptive recommendation systems.
Traditional machine learning models often rely on correlation-based patterns to make predictions, but causal discovery goes further by identifying the underlying cause-and-effect relationships within data. This more profound understanding of how variables influence each other makes models more accurate, interpretable, and reliable. When applied to recommendation systems, causal inference enables us to move beyond surface-level suggestions, offering insights that align with user behavior and system dynamics.
Our LSTM (Long Short-Term Memory) modeling expertise has already proven its value in predicting pod resource utilization in Kubernetes environments, as demonstrated by our research. Through LSTM, we developed an adaptive scaling model that optimized resource allocation in cloud computing, resulting in a 13.15% cost reduction and significant efficiency gains. But now, by incorporating causal discovery methods, we are taking predictive systems to the next level.
Causal machine learning offers transformative potential in industries like connected vehicle systems, smart cities, and telecommunications, where data complexity and interdependencies are high. Causal models can predict system behaviors more accurately, provide actionable insights, and generate more brilliant, personalized recommendations that adapt to real-time conditions. For instance, in V2X (Vehicle-to-Everything) architecture, these models can optimize traffic flow and improve safety, while in telecommunications, they can enhance network efficiency and user experience.
We are committed to leading the way in applying causal inference and discovery to AI-driven solutions. By developing models that not only predict but understand the causes behind the data, we are shaping a more innovative, more connected future across industries.
Our work continues to align with our core mission of providing innovative, data-driven solutions that empower businesses and contribute to sustainable growth. As causal machine learning becomes more integral to AI, we remain at the forefront, driving breakthroughs that enhance prediction accuracy, efficiency, and overall system intelligence.